Trust and machine learning AI in medical devices
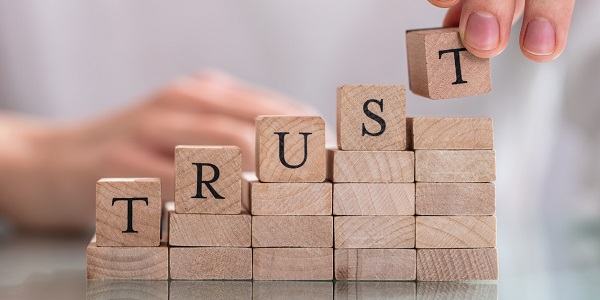
Machine learning AI moves beyond simply performing automated tasks and begins to edge into the practice of medicine
For more than 2,000 years, medicine has embraced the ethos of Hippocrates to “first, do no harm.” A corollary of that is any action taken to treat a disease, illness, or injury should alleviate the patient’s condition in some way—it must be effective. In modern times, ensuring the effectiveness of treatments has relied upon the use of the scientific method. Treatments and procedures must be scientifically established, must be supported by clinical evidence, and should be understood and explainable. Clinicians, who oversee these treatments and perform these procedures must show possession of high levels of scientific knowledge and technical skill and be licensed or accredited. In short, practitioners must demonstrate competence before they are permitted to practice medicine.
Trust[1] with respect to medical devices is different. Traditional rules-based medical devices do not practice medicine, but rather perform automated pre-programmed tasks. For medical devices and technology, acceptable adherence to the scientific method starts with using established scientific principles in device design, followed by conformance to consensus standards that require manufacturers to prove the effectiveness of their products through clinical investigations and empirical evidence, as well as compliance with governmental regulations. Those standards and regulations require that safety be demonstrated through testing and risk management. They also require manufacturers to employ various practices[2] in a quality management system that assure any substantive change to a product’s design, materials, manufacture, or function is similarly supported by clinical or empirical evidence. In other words, trust in medical technology is established not by demonstration of understanding and capability, but by validating that the technology produces reliable and predictable outputs.
Reliance on predicted outputs, however, may not work for machine learning AI, which moves beyond simply performing automated tasks and begins to edge into the practice of medicine. Utilizing the current practice of requiring a prior approval of any significant change, regulators will find it difficult to approve or clear a device for marketing if the rationale and evidence behind its actions are unclear or if the device’s performance and outputs change over time. Stakeholders (and the regulations and standards that support them) will need to find ways to expand beyond validated, predictable outputs and also consider competence if we are going to learn how to trust machine learning AI, as well as how much to trust it.
Learning to trust AI is proving to be a difficult task for society as a whole—popular fiction is replete with tales of machines that become self-aware and robots that rebel while some futurists warn us of AI’s dangers. This is not surprising—trust is derived from knowledge, and there is much we know we do not know about AI, as well as much we do not know we do not know.
If medical device regulators, clinicians, and patients are going to reap the benefits of machine learning AI, it is critical that an appropriate level of trust in these systems be established by a collaborative regulatory system. A lack of trust in AI could affect its acceptance; if machine learning AI technology is not used, clinicians and patients cannot benefit from the advances and efficiencies it offers. The need for trust is even greater with continuous learning models, where performance will change as more training data becomes available and the system refines itself. Users will naturally be suspicious of any system that gives differing results over time.
Conversely, there is danger in over-trusting AI—believing whatever the technology tells us, regardless of the performance limitations of the system. The propensity to trust too much is exacerbated by the current amount of hype that is setting unrealistically high expectations of the technology’s competence.[3]
Most people generally trust mature and complex technologies without completely understanding how they work or function. We fearlessly ride elevators without understanding the complicated system of brakes, counterweights, and safety cables that ensure the elevator cars do not fall, and we use our ATM cards without worrying that withdrawals are correctly recorded or that the banks’ computers are emptying our accounts. We trust these technologies not because we think there are no potential risks, but because we believe that these risks are adequately managed by the hidden controls incorporated into the system.
Such controls are not uniformly in place for machine learning AI, however, so the accuracy, safety, and performance of these systems cannot be assumed or taken as a matter of faith. While potentially capable of out-performing humans in terms of deriving correlations and patterns that we cannot empirically detect, machine learning systems do not currently demonstrate a similar ability to understand the contextual meaning of data. In linguistic terms, AI, being driven by formal programs and algorithms, is more adept at syntactic (logic and computational) learning than at semantic (meaning-based) learning.[4] Furthermore, the data sets used in AI learning systems are constrained—restricted either in terms of data sources or in terms of the types of data being processed.
The practical implication of these limitations is that data-driven AI systems are not always able to sufficiently evaluate their own base assumptions or to verify the quality of incoming data. They are, to some degree, fragile—they perform extraordinarily well when their base assumptions are solid and the data used is both accurate and relevant. If, however, there are even small errors or changes in this self-contained universe of assumptions and data, then the same systems can fail. AI systems are poor at handling the unknown-unknowns—they do not know what they do not know. Thus, any system that can learn can also mislearn—it can “acquire incorrect knowledge”[5] in a variety of ways.
[1] Several regulatory and standards efforts to define the “trustworthiness” of medical AI are underway. This post discusses the concept of trust/ trustworthiness but does not attempt to define these terms or to set specific requirements around them. To avoid possible conflict or confusion with those regulatory and standards efforts, the former term (“trust”) is used instead of the latter (“trustworthiness”) in this post.
[2] These quality system practices include but are not limited to design control, input verification, process and output validation, usability testing, and postmarket surveillance.
[3] “Gartner Says AI Technologies Will Be in Almost Every New Software Product by 2020” https://www. gartner.com/en/newsroom/ press-releases/2017-07-18gartner-says-ai-technologieswill-be-in-almost-every-newsoftware-product-by-2020
[4] For example, idioms and euphemisms are not meant to be taken literally and this presents challenges to Natural Language Processing (NLP) systems. For example, discussions about AI ethics may be “a hot potato” to readers of this paper, but that description would be confusing to NLP software. Humor and sarcasm are also artifacts of our everyday discussions but would be misunderstood by software.
[5] Adapted from the Merriam Webster definition of “Mislearn”
This is an excerpt from the BSI/AAMI white paper: Machine learning AI in medical devices: adapting regulatory frameworks and standards to ensure safety and performance. To browse our collection of medical device white papers, please visit the Insight page on the Compliance Navigator website.
Request more information today for a call back from a member of our sales team so that you can get a better understanding of how Compliance Navigator can meet your needs.
The Compliance Navigator blog is issued for information only. It does not constitute an official or agreed position of BSI Standards Ltd or of the BSI Notified Body. The views expressed are entirely those of the authors.