How is AI impacting device or system design?
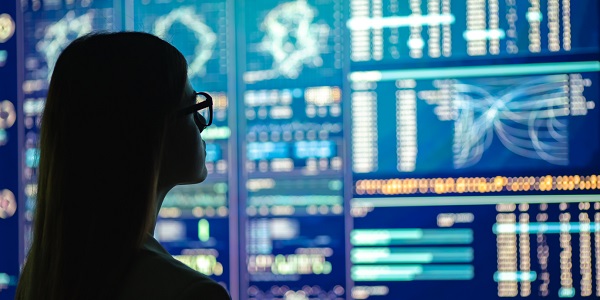
Data curation and review is becoming a key R&D activity for AI systems
One of the striking trends of the past 2 years – increased demand for employees with skill sets that include machine learning – might be attributed to the “Gartner hype-cycle”. However, a closer look suggests that there are genuine changes underway in the way systems are being engineered. These changes are seen across a wide range of institutions: in the activity of start-ups, within education and in large corporations. Much of this change is fuelled by the ease with which one can create, train and deploy software modules based on machine learning. But there is an underlying factor that is worth bearing in mind: as more data becomes available to train a modern machine learning system, the performance of the system – in terms of accuracy – gets better[1]. Thus, continual improvement has become an integral part of the attraction of machine learning in creating components of AI systems that read sensor data, text streams, and then adapt the overall behaviour of the AI system accordingly.
In the experience of this writer, there is increased investment into maintaining, as a core activity, teams who are responsible for data curation and performance monitoring. The dawning realisation is that to improve system performance, one should place strong emphasis on ensuring that data used to train a machine learning system are curated and managed. Let us take an example: for an AI module which is trained to detect an arrhythmia in electrocardiograms. Even after we have a system that has been deployed in a device, we would monitor and curate data that are acquired from many patients, identifying outliers in performance, identify incorrect decisions, and add these into the training set for the next generation of algorithm, perhaps with higher weighting. This becomes almost a process rather than an R&D activity, but it is valuable and important because good data curation and review processes have a very high probability of improving system performance within a predictable timescale: data has become a raw material in a very real sense.
The behaviour of autonomous systems might also be expected to follow similar patterns of development: improvements in technology performance will take several forms, but for systems that, say, perform diagnosis, performance improvements will take three main forms (a) reduction in false positives and negatives in detection subsystems (more accurate diagnosis); (b) reduction in cost of a device; and (c) improvements in power-efficiency/energy consumption, important for embedded controllers or implanted devices. The latter two of these will often require human expertise for the near term; the former becomes a process of data curation and retraining.
This is an excerpt from the BSI white paper Recent advancements in AI - implications for medical device technology and certification. To download our other medical device white papers, please visit the Insight page on the Compliance Navigator website.
[1] It is worth providing two minor caveats. First, this assumes that the capacity of the network, including the number of units and layers, has not been reached. If it has, the solution is simply to increase capacity: more layers, or more units. The second is that, at some point, there is simply no more available information in the data that would improve patient care; practically, we are seldom at this limit.
Request more information today for a call back from a member of our sales team so that you can get a better understanding of how Compliance Navigator can meet your needs.
The Compliance Navigator blog is issued for information only. It does not constitute an official or agreed position of BSI Standards Ltd or of the BSI Notified Body. The views expressed are entirely those of the authors.