AI: incorporation into medical devices and regulatory developments
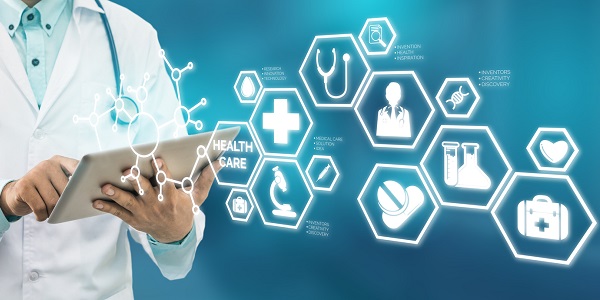
In this excerpt from the BSI medical devices white paper, Recent advancements in AI – implications for medical device technology and certification, Anil Anthony Bharath discusses drivers for the adoption of AI in medical devices and recent FDA regulatory activity
Adoption of artificial intelligence (AI) in medical devices
There are several drivers for the incorporation of AI into medical devices. Some of these might relate to marketing, and the attraction of AI-equipped systems to first-adopters. In areas where the quantity or complexity of data is high, including systems for intensive care monitoring, biomarker interpretation or visual diagnosis (e.g. MRI, dermatoscopes, ultrasound), assistance to a human interpreter can be provided by systems that can suggest diagnoses, or can retrieve cases which are in some sense, similar. This can support differential diagnosis, particularly for rare forms of some disorder; it is more than simply a retrieval system, as the criteria for matching is likely to be quite difficult to construct. Machine learning, informed by the actions of expert human diagnosticians, is likely to provide scalable and highly effective trained networks to disseminate expert knowledge and capability (Esteva et al., 2017).
The ability of systems based on machine learning to make use of ever increasing amounts of data, or, in this case, patient cases and their subsequent outcomes, is likely to drive improvements in performance – both in diagnosis and treatment planning and monitoring. Note, for example, significant research activity around machine learning for application in intensive care units (ICUs), with the aim of performing patient state or outcome prediction (Johnson et al., 2016). These efforts leverage the intensive and continuous recorded monitoring within such environments; with appropriate approaches to learning from wider electronic health records (Shickel et al., 2018), and long-term outcomes, the degree of improvements in sensitivity and specificity possible by AI is likely to yield diagnostic capabilities that are significantly more cost-effective than human teams for the same level of care.
With the use of reinforcement learning, it is also becoming possible to create autonomous agents – often built around deep neural networks – that are able to perform tasks that are extremely difficult for a human engineering team to code. Systems to recommend patient dosage (Chen, Zeng & Kosorok, 2016; Nemati, Ghassemi & Clifford, 2016) represent one example of the complex tasks – requiring previous case studies, patient interaction and response monitoring – that an autonomous agent can be trained to perform; see, for example, Tseng et al.’s work on dosage adaptation (Tseng et al., 2017).
Recent activity in the regulatory space
A number of pre-market approvals have recently been provided under the US Food and Drug Administration (FDA) “De Novo” pre-market pathway. The algorithm used in the Apple Watch AF detector is one such example. Diabetes management support tools that provide personalised treatment plans (DreaMed) represent another example.
The latter appears to use rather traditional “control-systems” based models of patients as its form of AI, and so – based on the published information – it is unclear to what extent machine learning is used. This is one example in which “AI” in a healthcare setting is unlikely to be using (at the time of writing) modern machine learning, where, for example, the model would implicitly be learned from examples of well-controlled patients.
Several recent FDA “De Novo” approvals are for image-based tools of AI. But the FDA has signalled a different approach that slightly shifts the centre of mass for certification – particularly for AI-related technologies – from a full focus on the “device” or its “performance”, to a focus on the company, and its processes. The FDA’s Digital Health Software Precertification Pilot Program programme was launched in 2019; in its current form, it leverages the idea of software as a medical device (SaMD), but pays particular attention to the culture of a company sponsoring the SaMD entity, including organizational excellence. This is a surprising move, but appears designed to support companies offering AI for patient management tools, diagnostic or analytical systems which arguably have no physical embodiment in the form of a device, but which could determine the course of treatment, and therefore carry life-or-death implications. A related move from the FDA is the “Breakthrough Device” designation, another pre-market process. Outcomes of public workshops and guidance on these schemes are available from the FDA website.
This is an excerpt from the white paper Recent advancements in AI - implications for medical device technology and certification, authored by Imperial College London's Anil Anthony Bharath. To download our other medical device white papers, please visit the Insight page on the Compliance Navigator website.
Request more information today for a call back from a member of our sales team so that you can get a better understanding of how Compliance Navigator can meet your needs.
The Compliance Navigator blog is issued for information only. It does not constitute an official or agreed position of BSI Standards Ltd or of the BSI Notified Body. The views expressed are entirely those of the authors.