AI and the Medical Devices Sector
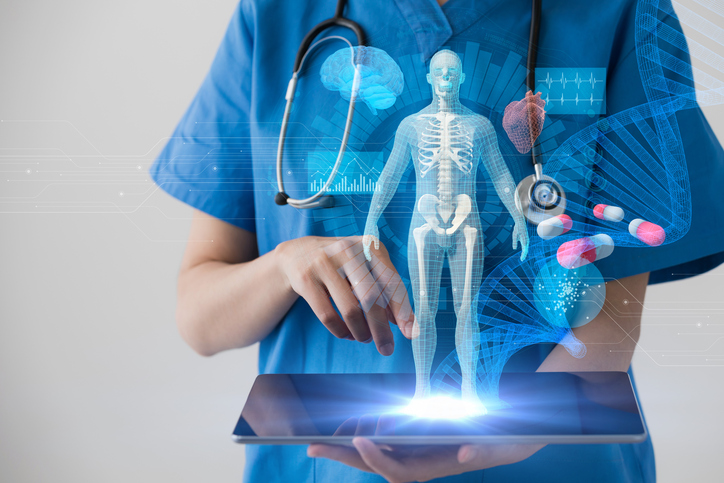
Artificial intelligence (AI) holds enormous promise for streamlining medical diagnostics, managing patient and clinical data, and contributing to research and development of medical devices. AI software is analogous to human learning and decision-making. This quality of learning, particularly inferential learning, provides the ability to learn without rule based programming and will allow researchers to model and evaluate all kinds of biological processes, at a speed that has not been possible before.
There are three areas of growth that are shaping how the technologies of AI, particularly deep learning inference, can assist clinicians and health care providers:
- Disease management – AI applications are being used to monitor both patient compliance with treatment regimens and the overall patterns of diseases in target populations. Wearable technology sends signals to patients that an action is required, such as medication, and sends a consequent signal to computers monitoring the treatment regime. Alerts are sent to clinicians or health care workers if a treatment pattern is interrupted or not followed. Algorithms are trained on high volumes of data so they can calibrate dosage based on demographics, disease stage and previous compliance history.
- Predictive analysis - The same data used for clinical management can be used to model disease clusters, patterns, and evolutionary pathways in populations when it is aggregated. An Australian government initiative in 2013 looking at chronic kidney disease. The yearlong pilot, which had 170,000 participants, resulted in a 300% increase in disease identification. The algorithm was built to identify at risk patients and order relevant screening tests for them. A similar study in the US in 2017 looked at the likely evolution of benign lesions into full blown breast cancer. The machine learning model was trained on 600 cases, relevant demographics and scientific literature and resulted in a 97% accuracy rate for predicting which lesions would become cancerous.
- Medical imagery – Medical imagery is one of the fastest growing areas for AI in the medical device sphere. IBM estimate that 90% of medical data is used for imaging and diagnostics. Uses of AI in medical imagery include:
- Ultrasound images to improve screening for cancers. In 2017, researchers working on detection of skin cancers tested an AI system detecting melanomas and found it to be 95% accurate, an 8.5% increase over dermatologist rates of accuracy. AI also misdiagnosed fewer benign moles as malignant melanoma. Scientists working in Japan have developed software which can detect colorectal cancer in its earliest stages with 86% accuracy. Colorectal cancer is often difficult to detect before it becomes deadly. Researchers in Houston have trained deep learning algorithms to create software which accurately models neck and head cancer tumours, allowing oncologists to calibrate radiation levels more precisely.
- Screening for macular degeneration. The Chinese government is investing in AI to assist with managing increasing levels of diabetic retinopathy and age related macular degeneration.
- Medical interpretation. Deep learning can help radiologists extract patterns and anomalies from thousands of images at a speed and with greater accuracy. AI can streamline workflows, improve image acquisition and sorting, and enhance image retrieval through sophisticated uses of the meta data associated with the images. In an effort to overcome some the computational issues of generating images, such as volume, white noise, or costs, researchers in America are working on ways to enhance existing images without having to collect additional data. Testing on machine learning platforms that can distinguish between high quality images and white noise images has begun at Massachusetts General Hospital. The AI platform is being trained to look for, and filter, properties of images, in addition to properties of normal or diseased pathologies.
Along with its potential, AI software brings many challenges, from cybersecurity to cost to classification. As the technology continues to evolve and its use expands into other areas of health, questions about when does software become a medical device and when it is technology that has a medical application will need to be answered.
The Compliance Navigator blog is issued for information only. It does not constitute an official or agreed position of BSI Standards Ltd or of the BSI Notified Body. The views expressed are entirely those of the authors.